Pearson’s Correlation Coefficient
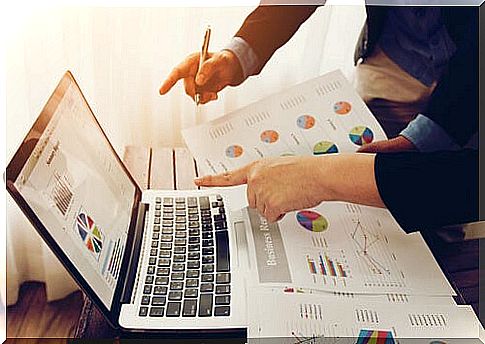
The correlation between two variables allows us to get an idea of the degree of association or covariation that exists between these two variables. Thus, the correlation coefficients are a kind of numerical representation of the relationship between the 2 variables (1). But what is Pearson’s correlation coefficient?
Bravais already made an approximation to what we know today as Pearson’s correlation coefficient in 1846. However, Karl Pearson was the first to describe, in 1896, the standard method of its calculation and to show that it is the best possible.
Pearson also offered some comments on an extension of the idea made by Galton. It was the latter who applied it to anthropometric data. Pearson called this method the “product moments” method (or the Galton function for the correlation coefficient r).
Person’s correlation coefficient is associated with the fit of very common models in statistics, such as regression analysis, its square -coefficient of determination- functioning as an indicator of goodness of fit.
Thus, Pearson himself (1896) spoke to us of the need for the variables analyzed (correlated, analyzed) to fulfill certain assumptions, such as normality.
On the other hand, in Spearman (1904) he noted:
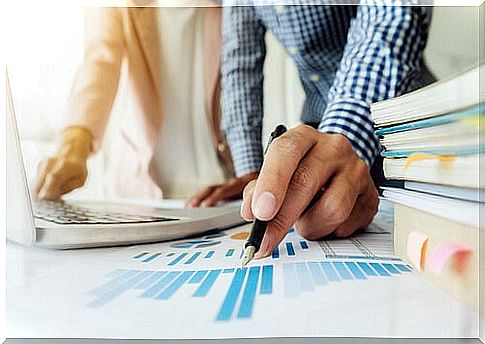
Spearman’s correlation coefficient and its function
Spearman’s correlation coefficient is a nonparametric rank statistic (with no associated probability distribution). It was proposed as a measure of the strength of the association between two variables. It is a measure of a monotonic association used when the distribution of data makes Pearson’s correlation coefficient misleading.
The Spearman coefficient is not a measure of the linear relationship between two variables, as some “statisticians” claim. Evaluate the degree to which an arbitrary monotonic function can describe the relationship between two variables.
Unlike Pearson’s correlation coefficient, it does not assume that the relationship between the variables is linear. It also does not require that variables be measured on interval scales; it can also be used for variables measured at the ordinal level.
In principle, the Spearman coefficient is simply a special case of the Pearson coefficient. In it, the data is converted to ranges before calculating the coefficient.
Assumptions underlying the correlation coefficient
The assumptions that support the Pearson correlation coefficient are the following (2):
- The joint distribution of the variables (X, Y) must be bivariate normal.
- In practical terms, to validate this assumption it must be observed that each variable is normally distributed. If only one of the variables deviates from normality, the joint distribution is not normal either.
- There must be a linear relationship between the variables (X, Y).
- For each value of X, there is a subpopulation of Y values normally distributed.
- Subpopulations of Y values have constant variance.
- The averages of the subpolations of Y are located on the same straight line.
- The subpopulations of X have constant variance.
- The means of the subpopulations of X lie on the same straight line.
- For each value of Y there is a subpolation of X values that are normally distributed.
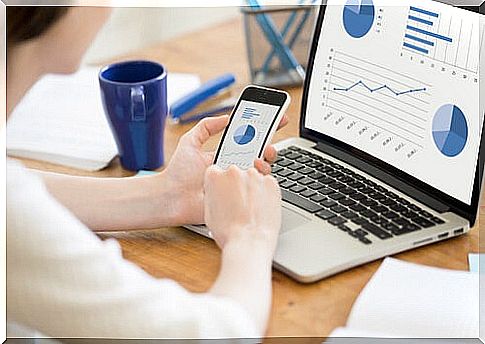
conclusion
Thus, when analyzing both Pearson and Spearman’s coefficients, one might expect that the meaning of one would imply the meaning of the other. On the other hand, a reverse implication does not necessarily appear to be logically true. Thus, the importance of the Spearman correlation can lead to the importance or not of the Pearson correlation coefficient. This occurs even for large data sets (1).
On the other hand, it is better not to use Spearman’s rank correlation coefficient as a measure of agreement, like the one we may need to calibrate an instrument. On the other hand, it is a very useful measure when we have many extreme values (the normality assumption is violated).